|
Christina B. Class, Otto Obert & Rainer Rehak
Are you looking for a little help from AI? These days, many software developers are doing just that. But how much can you trust generative tools? The following scenario illustrates how important it is to pose this question early on.
Three weeks ago, André was hired as a developer by Smart4All, a small firm specializing in custom software solutions for small to mid-sized companies. Recently, there has been an increased demand for AI-based services, both internally and externally, and André has been assigned to work in this area. He’s a newly minted BA who did reasonably well as a business and information technology major. At the moment, there is no shortage of IT job offerings. And yet, here he is—a guy whose strong suit wasn’t exactly statistics, programming, and AI—working in an IT department. Suffice it to say that while in school, he profited greatly from collaborations with his fellow students, especially in these areas.
He got lucky with his bachelor’s thesis: he completed the work at a mid-sized company where his job was to evaluate the potential for data mining and AI to minimize costs and optimize the preparation of proposals. After researching various blogs and on the code-sharing platform CoDev, André could find most of the code he needed. Then came the first version of Easy-AI-Code-Pilot—a tool for automatically generating code that was first introduced on CoDev. While he was skeptical at first, before long, he no longer had any qualms about using it all the time. It was no small task to get enough of a grip on the individual fragments to combine them to do what he wanted. At the time, the company was happy with his work and wrote him a glowing letter of recommendation.
Now, André is sitting at his new desk, staring out the window, when the team leader, Verena, comes in, smiles, and says she has an important assignment for him. BioRetail, a major nationwide distributor of organic products, has contracted Smart4All to develop new software solutions to integrate existing in-house programs for customer management, accounting, ordering, and warehousing. The client wants a solution to forecast incoming orders in a B2B format. There was data to prepare, processes to test, and everything to be documented in Python Notebooks…the usual. Verena flatters him—“That’s right up your alley!” she says. He subtly hints that these aren’t exactly his core competencies and that, while completing his bachelor’s degree, he’d relied primarily on such resources as blog entries and mainly used CoDev and Easy-AI-Code-Pilot to generate code. Verena grins at him and says, “That’s what everyone’s doing nowadays.”
André’s concerns thus fade, and he gets to work. Understanding and cleaning the data is no small feat for him, but he finds a snippet of code from a hackathon that involved scrubbing very similar types of data. He uses various code snippets culled from the internet that apply different cleaning methods. He tests the code snippets and evaluates them on the basis of the usual quality-control criteria. Easy-AI-Code-Pilot offers good suggestions for small subtasks, but André struggles to integrate all these different pieces of code. Even though there are times that he’s not quite sure of himself, in the end, everything looks plausible and consistent enough. However, he cannot guarantee that he may not have overlooked or incorrectly assigned one thing or another in all the data and code fragments. Nor did he adhere to any strict separation between training, validation and testing data sets. Time is ticking, and he brushes aside his creeping doubt because the results look convincing. He had, after all, run tests on various models using multiple hyperparameters for each one, and he correctly documented everything in Python Notebook files. He may not have come up with the perfect solution, but André is confident that the result is not too shabby.
Three weeks later, Verona and André are called in by Frederic, the IT product director and account executive for BioRetail. When they enter the room, they see Geraldine—the representative from BioRetail. The atmosphere is cold, and Frederic asks everyone to have a seat. Then Geraldine begins: at first, she was enthusiastic about the prognostic notebooks, but when she tried analyzing more recent data, it was spitting out results that made no sense whatsoever. Since the new data looked slightly different, she wanted to adjust the notebooks herself. That’s when she noticed that different program components were all based on different features. Then she saw even more inconsistencies, so she sat down with her IT people and reviewed everything. She couldn’t believe her eyes. The code was awful; it had zero homogeneity, and the data models were way too different. The documentation was atrocious. Everything was a hodgepodge slapped together from various methods that couldn’t function reliably. It was totally unacceptable, and any company capable of delivering such a slipshod product was certainly in no position to integrate multiple programs. Verena and Frederic exchanged glances before they looked at André and said: “So, you’ve got to have some explanation for this, right?”
Questions:
-
What is the value of using such tools as Easy-AI-Code-Pilot to generate code automatically?
-
What basic principles should be followed when using these assistance systems?
-
Should André have been more diligent about telling his team leader he wasn’t qualified to take on the assignment?
-
What was Verena’s role in this? As team leader, what should she have done better? Who is most at fault for the whole fiasco?
-
Does the platform provider CoDev have any responsibility for making a product like Easy-AI-Code-Pilot accessible free of charge? Is it enough for CoDev to display a text warning about potential product misuse?
-
What steps should providers of these kinds of tools take to live up to their obligations?
-
What do you think about Verena humiliating André in front of the IT product director and the BioRetail representative? What ethical principles should apply to management personnel in this situation?
-
Is it even possible to implement ethical principles in AI? What are the implications for the way we deal with AI? What might a code of ethics look like?
Published in .inf 05. Das Informatik-Magazin, Frühjahr 2024, https://inf.gi.de/05/gewissensbits-softwareentwicklung-mit-kollege-ki.
— Translated from German by Lillian M. Banks
Christina B. Class & Stefan Ullrich
A little over a year ago, Alex completed his master’s thesis on artificial intelligence and facial recognition. His customizable, self-learning method substantially improved previous results for real-time facial recognition. Last year, after he presented his paper at a conference—including a proof-of-concept live on stage—he was approached by the head of AI Research and Development at EmbraceTheFuture GmbH. The company was founded three years ago to specialize in the development of custom software systems, especially in the field of intelligence systems and security systems. After graduation, Alex took a short vacation and accepted a position working for EmbraceTheFuture GmbH.
He’s currently working in a small team to develop facial recognition software for a new security system called “QuickPicScan” that will be used at airports by the German Federal Police. The faces of passengers at security checkpoints will be compared in real-time with mugshots of fugitives so that suspicious individuals can be singled out and subjected to more intense scrutiny. Authorities hope that this will allow them to identify passengers with warrants within the Schengen area, where there are no passport controls at the borders.
It’s also designed to accelerate the rate at which people are processed through security checkpoints. The system was trained using millions of images. Mugshots and images of criminal suspects are stored in a database that is accessed and updated anytime a new image is captured so the system can easily be kept up-to-date with the most recent search warrants. At the airport, low-resolution photos of all passengers are taken as soon as they pass through security.
Whenever the software detects a match, the metal detector is triggered to sound the same alarm used when it detects metal. However, while the passenger is subject only to the routine search, a high-resolution photo is snapped under improved lighting. That image is again run through the system for potential matching. It isn’t until this second test produces a positive result that the passenger is taken aside and subjected to a more thorough search in a separate room where particulars are compared. The results of the second test are displayed on a control terminal. The photos of the passengers are not saved—there’s a separate team assigned to guarantee that these photos are deleted from the main memory and cannot be accessed externally. QuickPicScan was tested extensively in simulations and with actors in a studio set-up staged to replicate the security checkpoint.
Based on these tests, the team estimates a false negative rate of 1%. For every 100 people targeted for closer scrutiny, only one goes undetected. The false positive rate—the number of people incorrectly classified as suspicious—is less than 0.1%. Marketing director Sabine is delighted with these results. A margin of error of 0.1% for falsely targeted innocent subjects—that’s spectacular!
To test the system in real-world conditions, the company is coordinating with the police to conduct test runs for two months in the summer at a small airport—one that serves approximately 400,000 passengers per year. One of the client’s employees monitors the control terminal. Three hundred seventy actors were taken in “Mugshots” of varying degrees of quality and in various poses and fed into the system.
During the two-month testing period, the actors pass through the security checkpoint 1,500 times at previously determined randomly selected times. After passing through the checkpoint, they identify themselves at the control terminal so the system can be tested. Since the two-month period falls within the summer vacation, only 163,847 passengers are checked. The lamp incorrectly identifies 183 passengers as suspicious. Eight of the 1,500 security checks logged by actors failed to recognize the match.
Project manager Viktor is thrilled. While the false positive rate of 0.11% was slightly higher than initially hoped, the false negative rate of 0.53% was substantially lower than anticipated. EmbraceTheFuture GmbH goes to press with these numbers and a margin of error of 0.11%. The police announced that the system will soon be operational at a terminal in a major airport.
That evening, Alex gets together with one of his old school friends, Vera, who happens to be in town. She is a history and math teacher. After Vera has brought Alex up to speed on the latest developments in her life and love interests, he gushes to her about his project and tells her about the press conference. Vera’s reaction is rather critical—she’s not keen on automatic facial recognition. They’d often gotten into this while he completed his master’s degree. Alex is thrilled to tell her about how low the margins of error are, about the increased security and the potential for ferreting out individuals who’ve gone into hiding. Vera looks at him skeptically. She doesn’t consider the margin of error low. .11%? At a large airport, dozens of people will be singled out for closer inspection. And that is no laughing matter, in her view.
She also wonders how many people who’ve had their mugshots taken will likely be boarding a plane. But Alex doesn’t want to hear about it and goes on a tangent outlining details about the algorithm he developed as part of his master’s thesis…
A few months later, the system was installed at AirportCityTerminal. Security officials were trained to use it, and the press reported a successful launch. A couple of days later, Alex flies out of AirportCityTerminal. He’s already looking forward to passing through his QuickPicScan—basking in the knowledge that he has contributed to improving security. But the metal detector starts beeping no sooner than he goes through the security gate. He’s asked to stretch out his arms and place his feet on a stool—one after the other—all while staring straight ahead. He peers at the security guard’s screen to his right and sees the tiny light of the QuickPicScan monitor blinking. Let’s hope this doesn’t take long—he’s cutting it close with his flight. They won’t wait for him since he hasn’t checked any bags, and he can’t afford to miss this flight. He’s taken to a separate room and asked to keep his papers ready while he stands there opposite a security guard. Alex tries to give the guy his passport, but the guard tells him to wait—he’s not the one in charge, and his colleague will be by shortly to take care of it. Alex is growing impatient.
He asks them to confirm his identity and is told no—it can’t be done because the officer on duty doesn’t have access credentials for the new system. It takes a full eight minutes for the right person to show up. Once his identity has been confirmed, it’s clear that Alex is not a wanted fugitive.
But his bags are nevertheless subject to meticulous search. “It’s protocol,” the woman in charge tells him. Alex is getting antsy. He’s probably going to miss his flight. Suddenly, he’s reminded of the conversation he had with Vera.
“Does this happen a lot?” he asks, feigning politeness.
“A couple dozen a day, I suppose,” she says as she walks him back to the terminal.
Questions:
-
Alex was falsely identified as a “suspect” and missed his flight. This is referred to as a “false positive.” How much collateral damage from “false positives” will we take in stride? What kinds of fallout can falsely identified people be expected to accept? How would compensation for such instances be regulated?
-
People make mistakes, too. Under similar circumstances, it’s possible that Alex was singled out for closer inspection by a security agent. In principle, does it really make a difference whether it’s human error or machine error?
-
People are prejudiced. For example, it’s well known that men who appear to be foreigners are checked more frequently. What are the chances that software systems will reduce this type of discrimination?
-
Self-learning algorithms require training data, so their results are heavily dependent on training data. This could lead to built-in discrimination manifested in the algorithm itself.
-
It’s also conceivable, for example, that facial recognition for certain groups of people is less precise because fewer images of them are available in the training data. This may involve anything from skin color to age, gender, facial hair, etc. A system like the one presented here could lead to an excessive number of people with certain physical features being singled out for closer inspection. What can be done to eliminate the potential for discrimination in training data? How might systems be tested for discrimination?
-
Is there a conceptual difference between manifest discrimination built into a system and human discrimination? Which of the two is more easily identified?
-
People tend to readily trust software-generated solutions and relinquish personal responsibility. Does that make discrimination by technical systems all the more dangerous? What are the possibilities for raising awareness about these matters? Should consciousness-raising efforts be introduced to schools, and if so, what form should this take? Is that an integral component of digital competency for the future?
-
Figures for false positive and false negative rates are often given in percentages. So, margins of error under one percent don’t sound that bad at first glance. People frequently find it difficult to imagine how many individuals would be affected in real life and what the consequences and impact may be. The figures are often placed side by side without establishing the relationship between false positives (in our case, the number of people who show up in mugshots) and false negatives (in our case, the rest of the passengers). The ratio is often starkly unbalanced. In the test run described here, with a total of 163,847 people, 1,500 (positives) were identified, so about one in every 1,000 (1:1,000). Is this comparison misleading? Should these kinds of figures even show up in product descriptions and marketing brochures? Is it ethical for the responsible parties at EmraceTheFuture GmbH to go to press with this? Are there other means of measuring margins of error? How can the error rate be represented so systems can be realistically assessed?
Published in Informatik Spektrum 42(5), 2019, S. 367-369, doi : 10.1007/s00287-019-01213-x
— Translated from German by Lillian M. Banks
Christina B. Class & Debora Weber-Wulff
For years, they’ve been preparing for this. But now, 1950s-era dreams of a self-driving vehicle are finally coming true. They christened their creation “Galene”—the self-driving car. It performed like a champ on the test track. Even in test drives on American roads—for which Galene had to be shipped to the US—everything was swell. There were fewer regulations in the US, where endless highway stretches and good visibility combined allowed ample room for experimentation.
Everything was more complicated in Germany, and obtaining the necessary permits for testing on public thoroughfares took longer. The press has been invited to tomorrow’s widely publicized “maiden voyage.” Jürgen, one of Galene’s proud “parents,” has gotten approval from his team leader to take his baby out for a spin on the planned course before the press gaggle gets underway, just to be sure everything runs smoothly. He’s a good engineer, so his planning has been meticulous. It’s a Sunday afternoon when these roads won’t have much traffic. And he’ll be seated at the wheel himself to intervene should anything go wrong. He’s confident he won’t call attention to himself or annoy other drivers or passersby.
He tells the voice computer where he wants to go, and Galene confirms the destination. Then, he calculates the route, taking into account current traffic reports, known construction sites, and the weather forecast. Everything is good to go: there is no construction along the route, no rain, no fog, and only a slight breeze. It’s a sunny autumn day—perfect for the first test drive!
Jürgen is enjoying his ride along this route, which he knows well. It is a great feeling to let someone else do the driving, even though it still seems strange not to put on the gas, hit the brakes, or take the steering wheel. Galene enters the expressway flawlessly, passes a classic car, takes the next exit, slows to a crawl, and stops at the light. She always keeps her distance from the vehicle ahead of her. The steering is so precise that it could be set to approach within less than an inch of the car she is trailing. But that would put other drivers needlessly on edge, so Galene’s been programmed to maintain a distance of about 15 inches.
Jürgen would love to use his cell phone to film how he catches a “green wave” before he hangs a left at the third light—he wasn’t quite sure whether Galene would accurately calculate all the signals involved to make that happen, but she did: perfect! If he would pull out his phone and start filming, he could hardly sustain the illusion that he was the one driving the car. As they enter a newer residential district, Galene reduces her speed to the 30km/h limit. There’s a school on the left, with bus stops for school buses on both sides of the street. They invested a lot of time preparing Galene to deal with this type of traffic scenario.
Luckily, fall recess happens to be in session. To their right, they pass a park with sprawling grassy areas. He hears kids screaming and looks to his right. Jürgen sees dogs romping, brightly colored balls bouncing in the grass, and kites flying even brighter than that in the air. When the wind blows them in his direction, Jürgen instinctively grabs the steering wheel, knowing as he does that children at play ignore traffic.
Especially when he first started “driving” the self-driving car, this happened a lot: he would get nervous, reach for the steering wheel, and switch to manual control so he could take over. But he never needed to, so he gradually learned to relax and leave the driving to Galene. Now, though, suddenly, it happened: some kid with a kite in hand darted out onto the street from between two parked cars and was hit by Galene. The kid falls to the ground unconscious.
Galene immediately hits the brakes because her sensors have detected the impact. At the same time, Jürgen pulls the emergency stop button. Galene comes to a halt, and the hazard lights are activated. Jürgen gets out and runs toward the child, whose mother soon appears and starts going off on Jürgen. Another young woman exits the car, driving behind Jürgen, and begins administering first aid. She says she’s a nurse.
A dog owner visiting the park has already placed an emergency call,, and the ambulance arrives promptly to transport the child and his mother to the nearest hospital with blue lights flashing. The police are also on the scene to file an accident report. Jürgen appears to be in a state of shock. The young woman who administered first aid immediately approached the police, even before they had the chance to question Jürgen. She tells them her name is Sabine, and she was driving behind the vehicle involved in the crash. She thinks he was driving too fast. She was driving well below the 30 km/h speed limit—with all the sounds of kids playing in the park, the dogs chasing after balls, and the kites flying, you had to expect something like this to happen!
The police ask Jürgen for his license and registration. He gives them his ID, driver’s license, and the test drive permit. The cops are taken aback and start asking questions about the car—they’re intrigued. Since this involves a road test, but the vehicle is technically not licensed for operation on public roads, they insist on having Galene towed, mainly because the data had to be analyzed more thoroughly. Jürgen is sure that Galene followed the rules of the road, but the accusation made by the witness, Sabine, still weighs heavily on him. Tomorrow afternoon’s “maiden voyage” and press conference are in jeopardy. It’s a PR disaster—especially now after this accident.
Questions:
- The car had an official operating license for road tests. Was it okay to take it out for a test drive before the road test was completed?
- Airplane pilots are repeatedly required to undergo training to guarantee they can respond quickly in an emergency and take over controls from the autopilot. Will this type of training also be needed for self-driving cars? Should Jürgen have been permitted to sit back and relax during the road test?
- As soon as Jürgen saw children playing in the park, he instinctively grabbed the steering wheel. As a driver, should he be required to take control of the vehicle in a situation like this, where he could expect children to run into the street?
- Galene was following the 30 km/h speed limit, but the witness complained that this was too fast when there were so many kids playing in the park. When calculating speed, to what extent can and should algorithms account for activities along the roadway?
- Unforeseen events will always cause accidents, whether a child running out into the street, a wildlife crossing, or a tree branch down on the road. Disaster is often averted by a driver’s speedy response or instinctive hesitation. Should algorithms be programmed to emulate some instinct? To what extent can self-training systems be of use in this regard?
- Sometimes, rear-end collisions result from a driver following the rules of the road “too closely”: for example, stopping at a yellow light on a busy highway or sticking to the posted speed limit in the blind bend on an expressway exit ramp. Self-driving vehicles are programmed to adhere strictly to the rules. Should they be programmed with a built-in “bending of rules” based on the behavior of cars driving in front and behind them?
- It would be impossible to test for every imaginable scenario, so the software on a self-driving car may respond inappropriately. In that case, who is liable? The developer? The manufacturer? The driver who is seated at the wheel “just in case”? Or would we take these cases in stride in exchange for the greater security these cars provide in other circumstances? Where do we draw the line?
- How and when should software updates be installed for self-driving cars? Only at the dealership or wherever the vehicle happens to be located, as long as it is stationary. Who oversees and determines whether an update has been installed and when? What happens if an accident could have been prevented if a software update had been installed? And who is liable?
Published in Informatik Spektrum 38(6), 2015, S. 575–577.
— Translated from German by Lillian M. Banks
Stefan Ullrich, Reinhard Messerschmidt, Anton Frank
Von der Idee über die Datensammlung bis hin zur Nutzung sind oftmals viele verschiedene Menschen an einem Datenprojekt beteiligt. Dieses Fallbeispiel zeigt auf, welche moralischen Schwierigkeiten sich dabei eröffnen können.
Matilda, Micha und Meryem sind schon seit Schulzeiten befreundet und freuen sich daher sehr, dass sie ihr Freiwilliges Ökologisches Jahr (FÖJ) zu dritt bei einer gemeinwohlorientierten Organisation namens „Code Grün“ leisten können. Die Organisation arbeitet schon lange im „Civic Tech“-Bereich und möchte für ein gefördertes Projekt Daten für Umweltschutz sammeln. „Das ist doch etwas für ‚M hoch drei‘ – für uns!“, meint Matilda, schließlich haben sich die drei schon immer für die Natur interessiert.
Ganz konkret sollen sie in kleinen und mittleren Städten Temperatur und Luftqualität messen. Als sie die zahlreichen Messinstrumente sehen, kommt Meryem eine Idee: „Wir könnten die Sensoren in meinen Rollstuhl einbauen, da können wir auch ein paar Powerbanks einpacken!“ – „Ja, und für uns noch jede Menge Snacks und einen kleinen Kühlschrank“, witzelt Micha. Auch Matilda grinst und schlägt vor, neben den Umweltmessungen auch den Zustand der Barrierefreiheit mit zu erfassen, also die Funktionsweise von Aufzügen und den Zustand der Gehwege und so weiter.
Die ersten Stationen sind sie noch total enthusiastisch unterwegs, es ist spannend, so viele Gegenden im Umland kennenzulernen. Die Messung erfolgt mithilfe eines Sensor-Kits, das Code Grün zusammen mit einer Agentur entwickelt hat. Während ihrer Messfahrten fällt ihnen auf, wie sehr soziale Fragen mit Umweltschutzfragen zusammenhängen. „Durch die Stadtbäume hier ist es knapp vier Grad kühler als auf dem Marktplatz“, bemerkt Matilda, als sie im Hochsommer ihr wohlverdientes Eis unter einem Sonnenschirm genießen. „Na ja, den Leuten im klimatisierten Auto ist das ja egal“, kommentiert Micha. „Die ganzen abgesenkten Bordsteine und barrierefreien Straßen hier“, Meryem zeigt auf die kleinen Straßen und Gassen der Innenstadt, „sind für mich total praktisch, auch die Frau dort mit dem Kinderwagen kommt besser durch. Aber alles in der prallen Sonne, kein Wunder, dass das Baby schreit. Warum sind ausgerechnet hier keine Stadtbäume?“
Am Ende des Jahres wird es aber doch sehr zur Routine, der Spaß des Anfangs ist verflogen und ihnen wird bewusst, warum es eine FÖJ-Aufgabe ist. Um sich wieder zu motivieren, beschließen die drei, kleine Web-Apps zu schreiben, die auf ihren Daten basieren. Sie stellen sich dilettantisch an, nur Meryem hat bereits etwas Programmiererfahrung, aber es ist toll zu sehen, was man aus ihren Daten alles lesen kann. Auch die ganzen Ausreißer sind sehr amüsant, meistens Messfehler oder falsch angeschlossene Sensoren. Ihr Vorgesetzter bei Code Grün findet das spannend und bringt sie mit den „Devs“ zusammen, wie das Entwicklungsteam hier genannt wird. Mit den bereits vorhandenen Daten macht es sogar noch viel mehr Spaß, sich neue Web-Apps auszudenken.
In ihrem letzten Monat sollen die drei eine Präsentation vorbereiten und über ihre Datensammelei anekdotisch erzählen. Die Hauptrednerin des geplanten Events ist allerdings eine Person der Partner-Werbeagentur, die eine neue App vorstellen will. Die App ist toll gemacht, Umweltdaten werden erlebbar gemacht, mithilfe einer „Digitalen Lupe“ können Pflanzen und Insekten bestimmt werden und die „Zukunftslinse“ erlaubt Vorhersagen zur Luftqualität der kommenden Stunden und Tage.
Bei einer Sache werden Matilda, Micha und Meryem allerdings stutzig: Die App liefert auch eine Route für Personen im Rollstuhl mithilfe der Daten, die die drei erfasst haben. Erwähnt wird das allerdings nicht, es wird als „Prototyp basierend auf Daten von Code Grün“ vorgestellt.
Die ganze Idee mit der Barrierefreiheit war doch auf ihrem Mist gewachsen und nun schmückt sich eine Agentur mit fremden Federn! „Na ja“, sagt ihr Vorgesetzter am nächsten Tag, „die Daten sind ja für alle da, da können wir nicht jeden einzelnen Datensammler namentlich erwähnen. Die App ist außerdem auch kostenlos!“ – „Ja, kostenlos, aber nicht Open Source. Und die anderen Apps der Agentur werden teuer an Kommunen verkauft!“, ärgert sich Meryem.
Sie weiß nicht, warum sie die App der Agentur so stört, sie tut doch genau das, was sie soll, und ist sehr praktisch. Dennoch fühlt sie sich irgendwie ausgenutzt, obwohl alle sehr korrekt und offen waren. Sie kommt zur Einsicht, dass „M hoch drei“ ohne die Agentur niemals eine funktionsfähige App auf Tausende von Smartphones gebracht hätte. Vielleicht kann sie ihre Namen wenigstens noch in die Credits bekommen in einem zukünftigen Update.
Als einige Monate später die drei ihr Unbehagen fast schon wieder vergessen haben, stolpert Matilda zufällig über einen Online-Artikel, in dem erwähnt wird, dass der massiv gewachsene Datenpool des inzwischen ausgegründeten Start-ups in eine neuere App mit breiterer Funktionalität münden soll, an der mehrere Bundesländer bereits Interesse signalisiert haben. Eine Erfolgsgeschichte, oder?
Fragen:
- Es ist ein offenes Geheimnis, dass die Wertschöpfung der Daten selten bei den Entwickler*innen und eigentlich nie bei den Datensammler*innen stattfindet. Warum ist das ein ethisches Problem?
- Es ist nicht klar, dass die Idee mit der Barrierefreiheit wirklich vom FÖJ-Team stammt, es gibt ja auch andere Datenprojekte. Doch angenommen, es trifft zu: Wäre es angemessen, alle Datensammelnden namentlich zu erwähnen? Sollten sie auch als Ideengeber*innen benannt werden?
- Das aus der Agentur ausgegründete Start-up kann mit erheblichen Fördergeldern rechnen. Wie ist es in ethischer Hinsicht zu bewerten, dass der Datenpool von einem FÖJ-Team mit aufgebaut wurde?
- Was ändert sich aus ethischer Sicht, wenn Daten als digitale Gemeingüter öffentlich geteilt werden, und wie sollte deren kommerzielle Nutzung geregelt (oder gar ausgeschlossen) werden?
- Was für eine öffentlich-digitale Infrastruktur wäre nötig und was brauchen ihre Nutzer*innen, um solche Projekte gemeinwohlorientiert und ethisch reflektiert umsetzen, nachnutzen sowie längerfristig betreiben und weiterentwickeln zu können?
- Umweltschutz und soziale Fragen hängen zusammen, aber müssen diese Themen dennoch unterschiedlich in ethischer Hinsicht diskutiert werden?
Erschienen in .inf 06. Das Informatik-Magazin, Sommer 2024, https://inf.gi.de/06/gewissensbits-zwischen-wertschaetzung-und-wertschoepfung.
Christina B. Class, Otto Obert, Rainer Rehak
Ein bisschen Hilfe von der KI annehmen? Das machen heute viele in der Softwareentwicklung. Doch wie viel Vertrauen kann man den generativen Werkzeugen entgegenbringen? Ein Fallbeispiel zeigt, wie wichtig es ist, sich diese Frage frühzeitig zu stellen.
André hat vor drei Wochen als Entwickler bei Smart4All angefangen, einer kleinen Firma, die spezifische Softwarelösungen für kleine und mittelständische Unternehmen entwickelt. Seit kurzer Zeit werden hier auch intern und extern vermehrt Services basierend auf KI angefragt und in diesem Bereich soll er tätig sein. André hat vor Kurzem seinen Wirtschaftsinformatik-Bachelor ganz okay abgeschlossen. Aber zurzeit gibt es ja keine Probleme, eine IT-Stelle zu finden. Trotzdem fragt er sich, wie er denn gerade hier gelandet ist, denn Statistik, Programmieren und KI waren nicht unbedingt seine Stärken im Studium gewesen. Gerade in den Informatik-Fächern hatte er von Gruppenarbeiten profitiert.
Auch mit der Bachelorarbeit hatte André eher Glück. Er hat die Arbeit in einem mittelständischen Unternehmen angefertigt und sollte das Potenzial von Data Mining und KI zur Kostenminimierung und zur optimierten Angebotserstellung evaluieren. Nach etwas Recherche in diversen Blogs sowie auf der Code-Sharing-Plattform CoDev fand André viele brauchbare Code-Elemente. Dann kam noch die erste Version des Easy-AI-Code-Pilot auf CoDev heraus, ein Werkzeug zur automatischen Generierung von Code. Anfangs skeptisch, hatte er später keine Bedenken mehr, diesen sehr intensiv zu nutzen. Es war auch ganz schön anspruchsvoll, die einzelnen Fragmente gut genug zu verstehen, um sie dann für sein Vorhaben zu kombinieren. Die Firma war damals zufrieden und schrieb ihm eine sehr gute Referenz.
Nun sitzt André an seinem neuen Schreibtisch und starrt aus dem Fenster. Da kommt seine Teamleiterin Verena herein, lächelt und sagt, dass sie einen wichtigen Auftrag für ihn habe. BioRetail, ein großer überregionaler Verteiler von Bioprodukten, hätte Smart4All mit der Entwicklung einer neuen Softwarelösung beauftragt, die bestehende Eigenbau-Programme für Kundenmanagement, Buchhaltung, Bestellwesen und Lagerhaltung integrieren soll. Der Auftraggeber habe sich eine Lösung gewünscht, die den Bestelleingang in seinem B2B-Bereich prognostiziert. Es sollten Daten aufbereitet, Verfahren getestet und das Ganze wie üblich in Python-Notebooks dokumentiert werden. Das sei doch sein Spezialgebiet, schmeichelt ihm Verena. Vorsichtig deutet er Verena an, dass dies nicht unbedingt seine Kernkompetenzen seien und er in seiner Bachelorarbeit überwiegend auf Ressourcen wie Blogbeiträge und Code beispielsweise via CoDev und Easy-AI-Code-Pilot zurückgegriffen hätte. Verena grinst ihn an und meint, das sei heute doch völlig normal.
So schwinden Andrés Bedenken und er macht sich ans Werk. Die Daten zu verstehen und zu bereinigen ist nicht ganz einfach, aber er findet Codeschnipsel aus einem Hackathon-Wettbewerb, bei dem ganz ähnliche Datenarten bereinigt werden sollten. Er verwendet weitere Code-Segmente aus dem Netz für zusätzliche Verfahren, um diese dann zu testen und basierend auf gängigen Qualitätskriterien zu evaluieren. Der Easy-AI-Code-Pilot macht gute Vorschläge für kleine Teilaufgaben, aber André hat viel zu tun, um alle unterschiedlichen Codeteile zu integrieren. Obwohl er sich manchmal etwas unsicher ist, erscheint am Ende alles plausibel und konsistent. Ob er allerdings in all den Daten- und Codefragmenten nicht doch etwas übersehen oder falsch zugeordnet hat, kann er so schnell nicht feststellen. Auf eine strikte Trennung der Trainings-, Validierungs- und Testdaten hat er auch nicht geachtet. Die Zeit wird knapp und er wischt aufkommende Bedenken beiseite, da die Ergebnisse recht überzeugend wirken. Er hatte ja auch verschiedene Verfahren mit jeweils mehreren Hyperparametern getestet und alles in den Notebooks ordentlich dokumentiert. Selbst wenn er nicht die perfekte Lösung haben mag, ist sich André sicher, dass sich das Ergebnis sehen lassen kann.
Drei Wochen später werden Verena und er zu Frederic, dem Produktleiter IT und Kundenbetreuer von BioRetail gebeten. Als sie den Raum betreten, sehen sie Geraldine, die Vertreterin von BioRetail. Die Stimmung ist kühl und Frederic bittet alle, sich zu setzen. Dann fängt Geraldine an: Anfangs sei sie von den Prognose-Notebooks begeistert gewesen, aber als sie neuere Daten analysieren wollte, hätte es unsinnige Ergebnisse gegeben. Da die neueren Daten etwas anders aussähen, wollte sie selbst noch mal die Notebooks anpassen. Dabei sei ihr aufgefallen, dass die verschiedenen Programmteile auf unterschiedlichen Features basierten. Und dann habe sie noch weitere Inkonsistenzen bemerkt. Sie habe sich dann mit ihren IT-Leuten Zeit genommen, um alles in Ruhe anzusehen, und hätte ihren Augen nicht getraut. Der Code sei furchtbar, nicht aus einem Guss und die Datenmodelle zu unterschiedlich, die Dokumentation grauenhaft und alles ein Sammelsurium aus unterschiedlichen Ansätzen, die nicht verlässlich zusammen funktionieren könnten. Das sei inakzeptabel und eine Firma, die so etwas abliefere, sei auch nicht in der Lage, verschiedene Programme zu integrieren. Verena tauscht mit Frederic Blicke aus, bevor sie André anblickt und sagt: „So, du kannst das doch bestimmt erklären?“
Fragen:
- Wie ist der Einsatz von Werkzeugen wie Easy-AI-Code-Pilot zur automatischen Generierung von Code zu bewerten?
- Welche Grundprinzipien sind beim Einsatz eines solchen Assistenzsystems zu beachten?
- Hätte André gegenüber seiner Teamleiterin noch deutlicher darauf hinweisen müssen, dass er für die Bearbeitung der Aufgabe nicht ausreichend qualifiziert ist?
- Welche Verantwortung fällt Verena zu? Was hätte sie als Teamleiterin an welchen Stellen besser machen müssen? Wer trägt die Hauptverantwortung für das Desaster
- Welche Verantwortung fällt dem Anbieter der Plattform CoDev zu, einen solchen Easy-AI-Code-Pilot frei zur Verfügung zu stellen? Genügt es, wenn CoDev eine textliche Warnung bezüglich möglicher Fehlnutzung anzeigt?
- Wie sollten die Anbieter solcher Werkzeuge grundsätzlich agieren, um ihrer Verantwortung gerecht zu werden?
- Wie ist es zu bewerten, dass Verena André vor dem Produktleiter IT und der Vertreterin von BioRetail bloßstellt? Welche in dieser Situation anwendbaren ethischen Grundsätze sollten für Leitungspositionen gelten?
- Ist es möglich, ethische Prinzipien in KI zu implementieren? Was bedeutet das für unseren Umgang mit KI? Wie könnte eine Regulierung aussehen?
Erschienen in .inf 05. Das Informatik-Magazin, Frühjahr 2024, https://inf.gi.de/05/gewissensbits-softwareentwicklung-mit-kollege-ki.
Unser zweites Buch, Gewissensbisse – Fallbeispiele zu ethischen Problemen der Informatik, ist 2023 erschienen mit 50 ausgewählten Fallbeispielen. Diese wurden mit Schlagworten indiziert. Die Open Access Publikation wurde durch das Weizenbaum-Institut e. V. unterstützt.
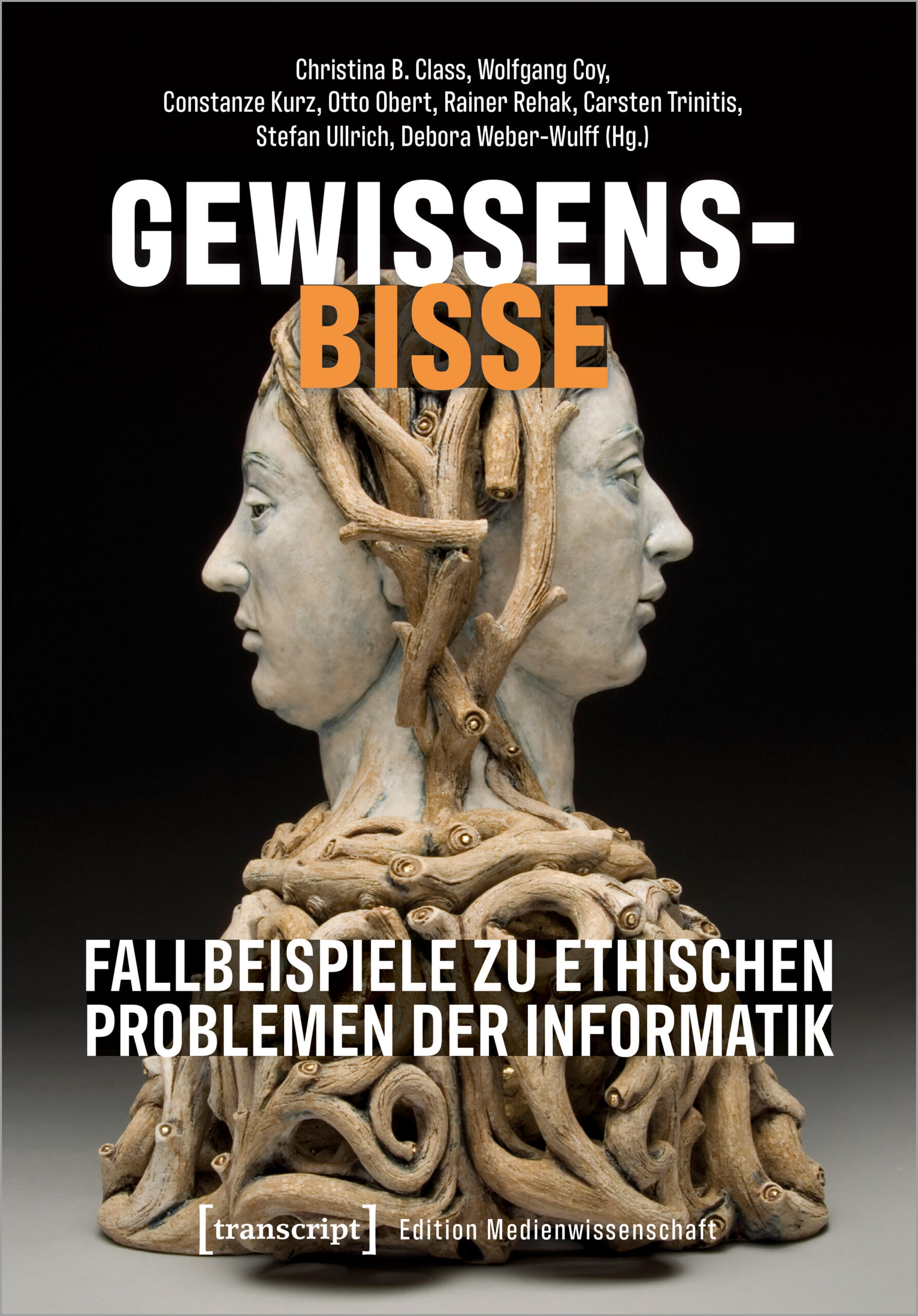 |
Abstrakt:
Die vielfältigen Möglichkeiten moderner IT-Systeme bringen drängende ethische Probleme mit sich. Neben der offensichtlichen Frage nach einer moralisch tragbaren Verwendung von Informationstechnologien sind ebenso die Aspekte des Entwerfens, Herstellens und Betreibens derselben entscheidend. Die Beiträge setzen sich mit dem Konfliktpotenzial zwischen Technik und Ethik auseinander, indem sie lebensnahe Fallbeispiele vorstellen und fragenbasiert zur Diskussion einladen. Damit liefern sie eine praktische Herangehensweise zum gemeinsamen Nachdenken über moralische Gebote und ethischen Umgang mit IT-Systemen und ihren Möglichkeiten. Der Band eignet sich damit in hervorragender Weise zum Vermitteln und Erlernen von ethischer Reflexions- und Handlungskompetenz in der Informatik sowie im Umgang mit IT-Technologien überhaupt. |
Class, C. B., Coy, W., Kurz, C., Obert, O., Rehak, R., Trinitis, C., Ullrich, S., & Weber-Wulff, D. (Eds.). (2023). Gewissensbisse—Fallbeispiele zu ethischen Problemen der Informatik. transcript Verlag. https://doi.org/10.14361/9783839464632
Gebundenes Buch: 29. Juni 2023, 240 Seiten, ISBN: 978-3-8376-6463-8
Digitalfassung: 6. Juli 2023, 240 Seiten, ISBN: 978-3-8394-6463-2, Dateigröße: 2.46 MB
Debora Weber-Wulff, Constanze Kurz
In kirchlichen Gemeinden fehlt es heutzutage oft an Menschen, die Seelsorge leisten können. Kann eine KI hier die Lösung sein?
Im ländlichen Raum spitzt sich die Lage immer mehr zu: Es gibt kaum Pastorinnen oder Pastoren, die seelsorgerisch tätig sind. Das Start-up KI-Talks will daher eine KI mit einem besonderen Textkorpus trainieren, sodass diese in der Seelsorge eingesetzt werden kann.
Matthias, der Geschäftsführer von KI-Talks, ist bei einem Team-Meeting voller Enthusiasmus. „Wir können die schon vortrainierte KI LLaMA von Meta nehmen und sie mit bestimmten Texten weiter trainieren. Also nicht nur mit Texten aus der Bibel und viel theologischer Literatur – wir könnten auch gleich alle Predigten, die im Internet auf Deutsch zu finden sind, in die Trainingsdaten geben.“ Er denkt außerdem darüber nach, ob es sinnvoll wäre, ältere Bibelübersetzungen hinzuzunehmen.
Oktay, Senior Engineer bei KI-Talks, fragt in die Runde, warum es denn eigentlich nur christliche Texte sein sollen. Sollte nicht auch der Koran hinein? Und die vielen jüdischen Auslegungen der Tora? Er fragt auch: „Sollten wir nicht gleich mehrere verschiedene LLaMAs trainieren? So können die Leute auswählen, welches sie haben wollen. Vielleicht gibt es sogar einen Vergleichsmodus, wo man sehen kann, was der Iman meint, was der Rabbi oder was die Pastorin?“
Emma, Frontend Engineer bei KI-Talks, schnaubt: „Ihr glaubt wohl, es gibt nur eine Auslegung? Was ist mit feministischer Theologie? Und dann müssen wir auch die ganzen Fundamentalisten mit ihren wortgetreuen Bibelauslegungen beachten: Sollen die auch abgebildet werden? Und kann LLaMA die wirklich alle auseinanderhalten? Es gibt auch weitere Religionsunterschiede: Ich glaube, die Katholiken sehen die Welt ein bisschen anders als die Lutheraner.“
Matthias wirft ein, dass man die Varianten sehr wohl unterscheiden könne. Ähnlich wie beim Segensroboter BlessU-2 [1], bei dem man die Art des Segens (Ermutigung, Erneuerung, Begleitung oder traditionell) auswählen kann, sollte man zum Beginn des Gesprächs einfach auf den gewünschten Religionsknopf klicken.
Emma meint, es sei überhaupt nicht klar, ob man verschiedene Religionsvarianten automatisch auseinanderhalten kann. Gerade bei der Unterscheidung von Fundamentalismus und weniger radikalen Auslegungen werde ein Knopfdruck nicht ausreichen, da brauche man eher einen Schieberegler. Auf jeden Fall müsse man erst einmal ausprobieren, ob so etwas überhaupt möglich sei.
Wo sei da eigentlich die Grenze, fragt sie noch, bevor Matthias versucht zu besänftigen. Er wirft ein: „Leute, mal das alles beiseite, habt ihr im Studium etwa nichts über Eliza von Joseph Weizenbaum gelernt? Das ist doch der erste Chatbot gewesen! Eliza hat Psychoanalytik betrieben – im Grunde ist das nur Zuhören und Gesagtes an den Menschen zurückspiegeln. Mehr ist Seelsorge auch nicht, nur ein bisschen zuhören, so tun, als ob man versteht, und damit die Menschen dazu bringen, selber einzusehen, was sie nun tun sollen.“
Emma kann sich zwar erinnern, dass im Studium auch etwas Kritisches zu Eliza gesagt worden war, aber sie weiß nicht mehr genau, was das war. Sie wirft noch ein, dass die Firma bereits so etwas Ähnliches gemacht hat: Es gibt doch deren KI-gestützten persönlichen Coach. Vielleicht könnten sie auch die Tests von damals verwenden. Aber sie besteht darauf, eine sehr breite Basis an theologischen Texten für das Training zu verwenden.
Oktay möchte viel Zeit in das Testen investieren, denn er glaubt, dass es problematische Gespräche geben kann. Man müsse viel Feedback von Menschen sammeln, die mit dem Chatbot sprechen, um zu verhindern, dass sich jemand nach einem Gespräch vielleicht etwas antut. Das ist schon bei anderen Chatbots passiert. [2]
Matthias sieht keinen Sinn in zu vielen Tests. Das kann man in späteren Versionen nachjustieren. „Wir müssen nur zuerst am Markt sein, dann können wir Zeit in Nachbesserungen stecken“, sagt er. Er entwirft nun einen straffen Zeitplan, denn er ist sich sicher: Auf den Chatbot von KI-Talks hat die Welt gewartet. Man müsse sich schon deswegen beeilen, weil die seelsorgerische Not mit jedem Tag wachse. Und ein gutes Geschäft könne man dabei sicher auch machen. Er wird gleich morgen einen Termin mit der Marketingfirma vereinbaren, die sie bei der Markteinführung unterstützen könnte.
Fragen:
- Ist es ein ethisches Problem, dass Matthias, Oktay und Emma beruflich einen Chatbot für die Seelsorge trainieren und programmieren wollen, obwohl offenbar niemand plant, Fachleute für Seelsorge oder Religion hinzuzuziehen?
- Ist es ethisch problematisch, wenn man mit einer bot-basierten Technologie Gewinn aus den seelsorgerischen Nöten der Menschen erzielen will?
- Müssen technische Systeme für Seelsorge mit besonderer Sorgfalt bedacht werden? Warum oder warum nicht?
- Ist es ethisch vertretbar, überhaupt einen Seelsorge-Chatbot erstellen zu lassen? Macht es einen Unterschied, ob man mit einem Menschen kommuniziert oder einem Bot?
- Sollten seelsorgerische Anwendungen kontrolliert werden, wenn sie potenziell eine Gefahr für Menschen darstellen könnten? Ist das überhaupt möglich?
- Wie könnte die Qualität von solchen Chatbots getestet und kontrolliert werden?
Quellen:
[1] https://www.youtube.com/watch?v=XfbrdCQiRvE sowie ein Artikel, der leider nur noch im Internet-Archiv verfügbar ist
[2] https://de.euronews.com/next/2023/04/02/chatbot-eliza-ki-selbstmord-belgien
Erschienen in .inf 04. Das Informatik-Magazin, Winter 2023, https://inf.gi.de/04/gewissensbits-im-seelsorgerischen-ki-gespraech
Carsten Trinitis & Anton Frank
IT can work wonders, but it can also become a weapon of war. This raises difficult moral questions, especially when it comes to choosing an employer.
Johanna is a student at a renowned university in southern Germany, where she has just defended her master’s thesis in computer science. Her thesis deals with automatic image recognition in poor weather conditions using machine learning.
From early childhood on, her parents raised her to handle nature with care, so she is absolutely euphoric over the fact that she is able to apply what she learned at university and in her master’s thesis to environmental protection. She has already been in contact with a start-up that specializes in early detection of forest damage aided by AI-controlled drones.
Recently, however, Johanna received an extremely lucrative job offer from a company in southern Germany that specializes in image recognition and automatic control of military drones. Even though the job sounds very appealing and is closely related to the topic of her master’s thesis, she rejects the offer outright because she hails from a pacifist family. Her parents demonstrated with prominent members of the peace movement back in the 1980s. They certainly would never talk to her again if she took a job in the arms industry!
At the commencement ceremonies for her graduating class, Johanna meets fellow student Volodymyr, who has been studying at the same school for two semesters. He’s really interested in her research because he also wants to specialize in the same field and has already completed an internship working on the military use of drones. Volodymyr had to leave his original university after five semesters because his home country was attacked by a neighboring country and regular instruction was no longer possible. He invites Johanna to come to the weekly get-together of his fellow refugees.
There she meets Julija and Oleksandr, who tell her about their family situations and the dangers they and their compatriots who have fled their homes still face to this day. The unspeakable suffering and dangers of the conflict there become more real and slowly take on names and faces for Johanna. Over the course of the evening, Johanna is increasingly confronted with the accusation that her country is not providing enough support, including in the military sphere. Her pacifist arguments fall on deaf ears, and she is told in detail how much less suffering would be caused if the invaded country were equipped with the appropriate reconnaissance and defense technology.
Johanna is reminded of the offer from the military technology company that she’s already turned down. After much hesitation, Johanna is just about to tell the others about the job offer when Alexei joins the group. He, too, was recently forced to flee his home country because he tried to speak up for the LGBTQ community. His country is the one that attacked Volodymyr’s. He talks about his parents who are living in the border zone and the way a neighbor’s house was recently destroyed in a drone attack that killed the father of the family living there. Following a brief round of goodbyes, Johanna—visibly shaken—makes her way home. She rifles through the mailbox, looking for the job offer, and reads it again. It just leaves her filled with cluelessness and despair.
Authors’ note: We want to make this clear—we know that there are political answers to many of the questions posed here. We, however, are strictly concerned with the ethical dimensions of these questions.
Questions:
- Should Johanna follow her parents’ lead and dismiss out of hand any job that involves military production?
- What does it take to have a clear conscience about the environment? Is it enough to place your own personal research in the service of environmental protection?
- How should Johanna act towards Volodymyr? Should she share with him her research results even if she knows he may use them for military purposes?
- Is military aid to protect the people in Volodymyr’s homeland justified, even if it potentially endangers Alexei’s parents?
- In this case, wouldn’t it be better to do everything humanly possible to help the invaded country?
- How far should this aid go—humanitarian, military, …?
- How do we assess the ethics of a technology that may be designed primarily for the purpose of protecting human life, but which might also lead to people being killed?
- Would research into military solutions be justified if Johanna were able to guarantee that such drones were used exclusively for defense purposes and not for attacks?
- Should research into dual-use goods be dismissed altogether simply because the potential for misuse exists?
Published in .inf 03. Das Informatik-Magazin, Fall 2023, https://inf.gi.de/03/gewissensbits-wenn-wissen-zur-waffe-wird
— Translated from German by Lillian M. Banks
Carsten Trinitis und Anton Frank
Informatik bewirkt viel Gutes, sie kann aber auch zur Waffe werden. Gerade bei der Wahl des Arbeitgebers stellen sich daher schwierige moralische Fragen.
Johanna studiert an einer renommierten süddeutschen Universität und hat gerade ihre Masterarbeit im Fach Informatik erfolgreich verteidigt. Darin hat sie sich mit dem Thema automatische Bilderkennung unter schlechten Wetterbedingungen mithilfe von maschinellem Lernen beschäftigt.
Weil sie von ihren Eltern seit ihrer Kindheit zu einem sorgsamen Umgang mit der Natur erzogen wurde, freut sie sich voller Euphorie darauf, das in Studium und Masterarbeit erworbene Wissen im Bereich des Umweltschutzes einsetzen zu können. Hierzu ist sie bereits in Kontakt mit einem Start-up, das sich auf die Früherkennung von Waldschäden mithilfe von automatischen, KI-gesteuerten Drohnen spezialisiert hat.
Kürzlich hat Johanna allerdings noch ein äußerst lukratives Jobangebot einer Firma aus dem süddeutschen Raum erhalten, die sich auf Bilderkennung und automatische Steuerung von militärischen Drohnen spezialisiert hat. Auch wenn die Aufgabe sehr reizvoll klingt und inhaltlich sehr eng mit ihrer Masterarbeit verbunden ist, verwirft sie dieses Angebot sofort, da sie aus einem pazifistischen Elternhaus stammt. Ihre Eltern haben bereits in den Achtzigern mit prominenten Mitgliedern der Friedensbewegung demonstriert. Sie würden bestimmt nicht mehr mit ihr reden, wenn sie einen Job in der Rüstungsindustrie annehmen würde!
Auf der Abschlussfeier ihres Semesterjahrgangs lernt Johanna ihren Kommilitonen Volodymyr kennen, der seit zwei Semestern an ihrer Universität studiert. Er zeigt sich sehr interessiert an ihren Forschungen, weil er sich ebenfalls auf dieses Thema spezialisieren möchte und sich in einem Praktikum bereits mit dem militärischen Einsatz von Drohnen beschäftigt hat. Volodymyr musste nach fünf Semestern seine Universität verlassen, da sein Heimatland vom Nachbarland angegriffen wurde und kein regulärer Lehrbetrieb mehr möglich war. Er lädt Johanna ein, zum wöchentlichen Stammtisch seiner geflüchteten Landsleute zu kommen.
Dort lernt sie Julija und Oleksandr kennen, die ihr von der Situation ihrer Familien und den Gefahren, denen die Familien der geflüchteten Landsleute immer noch ausgesetzt sind, erzählen. Das unsägliche Leid und die Gefahren des dort herrschenden Konflikts werden realer und bekommen für Johanna langsam Namen und Gesichter. Im Laufe des Abends sieht sich Johanna zunehmend mit dem Vorwurf konfrontiert, dass ihr Land nicht genügend Unterstützung leiste, auch im militärischen Bereich. Ihre pazifistische Argumentation stößt auf taube Ohren, und man erläutert ihr ausführlich, wie viel weniger Leid doch entstehen würde, wenn das überfallene Land mit entsprechender Aufklärungs- und Abwehrtechnik ausgestattet wäre.
Da kommt Johanna das bereits verworfene Angebot der Militärtechnikfirma wieder in den Sinn. Als sie nach langem Zögern schon darüber nachdenkt, den anderen davon zu erzählen, stößt Alexej zu der Runde. Auch er musste kürzlich aus seinem Heimatland fliehen – dem Land, das das Heimatland von Volodymyr angegriffen hat. Alexej musste jedoch fliehen, weil er versucht hat, der dortigen LGBT-Community eine Stimme zu geben. Er erzählt von seinen Eltern, die im Grenzgebiet wohnen und dass dort erst kürzlich eine abgeschossene Drohne das Haus der Nachbarn zerstört und den Familienvater getötet hat. Nach einer kurzen Verabschiedung geht Johanna sichtlich verstört nach Hause. Sie durchsucht ihr Postfach nach der Mail mit dem Jobangebot und liest es sich noch mal durch. Bei ihr machen sich Ratlosigkeit und Verzweiflung breit.
Anmerkung der Autoren: Wir möchten klarstellen, dass viele der gestellten Fragen auch politisch beantwortet werden können. Uns geht es hier aber einzig und allein um die ethische Dimension dieser Fragen.
Fragen:
- Soll Johanna sich an ihren Eltern orientieren und konsequent ablehnen, an militärischen Produkten zu arbeiten?
- Reicht es aus, die eigene Forschung in den Dienst des Umweltschutzes zu stellen, um ein reines Gewissen zu haben?
- Wie soll sich Johanna ihrem Kommilitonen gegenüber verhalten? Ist es richtig, ihre Forschungsarbeiten mit ihm zu teilen, auch wenn es ihm ermöglicht, sie für militärische Zwecke einzusetzen?
- Ist die militärtechnische Hilfe zum Schutz der Menschen in Volodymyrs Heimatland gerechtfertigt, auch wenn diese Alexejs Eltern potenziell gefährdet?
- Ist es in diesem Fall doch besser, alles Erdenkliche zu tun, um dem überfallenen Land zu helfen?
- Wie weit soll diese Hilfe gehen – humanitär, militärisch, …?
- Wie ist eine Technologie ethisch zu bewerten, die zwar primär der Verteidigung und damit dem Schutz von Menschenleben dient, trotzdem aber auch dazu führen kann, dass Menschen getötet werden?
- Wäre die Erforschung militärtechnischer Lösungen zu rechtfertigen, wenn Johanna sicherstellen kann, dass solche Drohnen ausschließlich zur Verteidigung und nicht zum Angriff genutzt werden können?
- Ist Forschung an Gütern mit doppeltem Verwendungszweck (dual use goods) wegen einer möglicherweise missbräuchlichen Verwendung grundsätzlich abzulehnen?
Erschienen in .inf 03. Das Informatik-Magazin, Herbst 2023, https://inf.gi.de/03/gewissensbits-wenn-wissen-zur-waffe-wird
|
|
Kommentare